
AI in action: assisting our client in building a custom RAG chatbot
In the vast and ever-evolving landscape of e-learning, the challenge of managing and utilizing extensive content libraries effectively is a common hurdle. At Panenco, we recently tackled this challenge head-on for one of our clients, who is a prominent player in the e-learning industry, by building a custom Retrieval-Augmented Generation (RAG) chatbot. The core design of our RAG chatbot solution emphasizes flexibility, enabling seamless adaptation and integration across diverse contexts, thus ensuring that it can be tailored to enhance content accessibility and utilization, irrespective of the domain or industry.
The challenge: overwhelming content and repetitive support queries
In this use case, our client possessed an extensive and ever-growing library of educational materials. This resulted in users often feeling overwhelmed by the sheer volume of available courses, tutorials, projects... Additionally, the client's support team was inundated with repetitive queries, an issue that significantly impacted the support team’s efficiency. These challenges underscored the need for a smarter, more intuitive way to guide users through the content and address their queries.
Customizing the RAG chatbot solution
In response to this challenge, we built, designed and implemented a RAG chatbot. This AI assistant leverages user-specific data, such as completed courses and other history, together with advanced reasoning built on top of OpenAI’s Large Language Models (LLM) to provide personalized content recommendations and support.
Key functionalities of this chatbot included:
1. Personalized content navigation: by analyzing the user's history and preferences, the chatbot could effectively guide them through the learning materials, suggesting courses and resources tailored to their educational journey.
2. Efficient query resolution: the chatbot was equipped to search through a comprehensive database of support articles, offering precise and timely answers to user queries, thereby reducing the repetitive load on the support team.
Engagement and interaction: a user-centric approach
To ensure the chatbot's effectiveness, we implemented various user engagement strategies. These ranged from context-aware messages to interactive prompts on key pages, all designed to facilitate a seamless and engaging user experience. Our focus was on making the chatbot not just a tool, but an intuitive and responsive guide to the user’s learning journey.
Continuous improvement through feedback
The development of this chatbot was an iterative process, heavily reliant on user feedback and performance metrics. We closely monitored user interactions, gauging satisfaction and identifying areas for improvement. These areas were steadily turned into test cases, extending the automated chatbot test suite to ensure that the AI assistant responds as expected and does not return broken URLs. This feedback loop was crucial for refining the chatbot, ensuring it remained performant, efficient, and never regressed.
Conclusion: tailoring AI for specific client needs
With engagement going up quickly, while increasing customer satisfaction scores to in the nineties, we enabled users to find relevant content quicker and get the proper answers to their questions. This success story is just one example among numerous AI-powered solutions that we've developed in response to evolving customer requirements, particularly in the realm of generative AI. Feel free to check out our website to learn more about these!

See also
Are you looking for an entrepreneurial digital partner? Reach out to hello@panenco.com or schedule a call
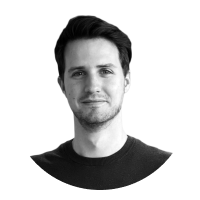
